Language of Molecules: Way to Accelerate Material and Drug Discovery
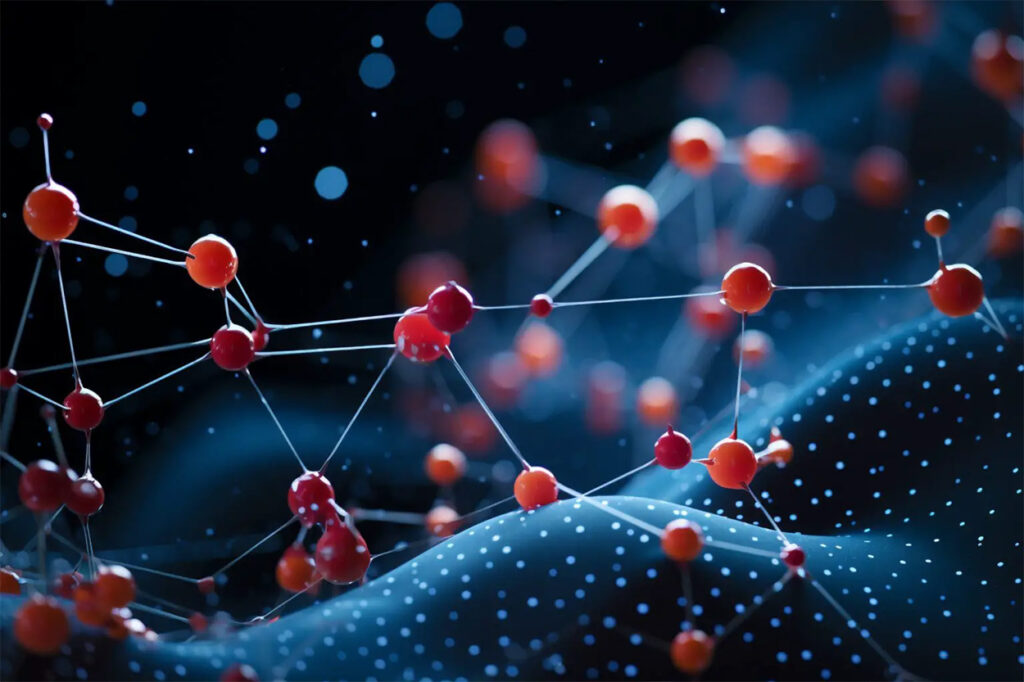
On average, developing a new drug takes between ten and fifteen years, involving three main phases. First is drug discovery, wherein the candidate compounds are chosen according to the required pharmacological properties. Second is the preclinical development, and third is the clinical development.
In the drug discovery phase, scientists identify the target, a protein associated with a specific human disease that the new drug intends to remedy or cure. Several tests follow to ensure candidate molecules will bind better to the target. Optimization continues to determine how the candidate molecules perform in the body. The scientists will also study drug absorption, metabolism, distribution, elimination, etc. This initial development phase takes between two to five years.
The new method from MIT
The drug discovery phase becomes easier with the system developed by the MIT-Watson AI Lab. With the help of artificial intelligence, the system simplifies drug and material discovery. It can accurately predict molecular properties even if the available data is minimal. MIT’s system utilizes a molecular grammar it learns through reinforcement learning to efficiently produce new molecules even if less than 100 samples are in a dataset.
Discovering new materials and drugs is a painstaking process as it passes through a manual, trial-and-error procedure that costs millions of dollars and long years of work. Many scientists today use machine learning to streamline the process of predicting molecular properties and minimize the molecules they must synthesize and test.
But with the new, unified framework the MIT and the MIT-Watson AI Lab developed, they can now simultaneously calculate molecular properties and produce new molecules more efficiently than the deep-learning methods popular today.
Machine learning approaches might be helpful, but it takes a long time to train the machines, and they need millions of hand-labeled structures and large training datasets, which are often difficult to obtain. Thus, the effectiveness of machine learning becomes limited.
Faster method
The system developed by MIT researchers can work effectively using a small amount of data, operating on the interrelated understanding of the rules that prescribe how building blocks combine to create valid molecules. The system’s rules acquire the similarities between molecular structures to help it create new molecules and determine their properties efficiently based on data.
The researchers used small and large datasets and discovered that their system outperformed most machine-learning methods. According to the study’s lead author Minghao Guo, their objective is to use data-driven methods to discover new molecules faster, so they can train a model to predict without using costly experiments. They will present their research at the International Conference for Machine Learning in Hawaii in the last week of July 2023.
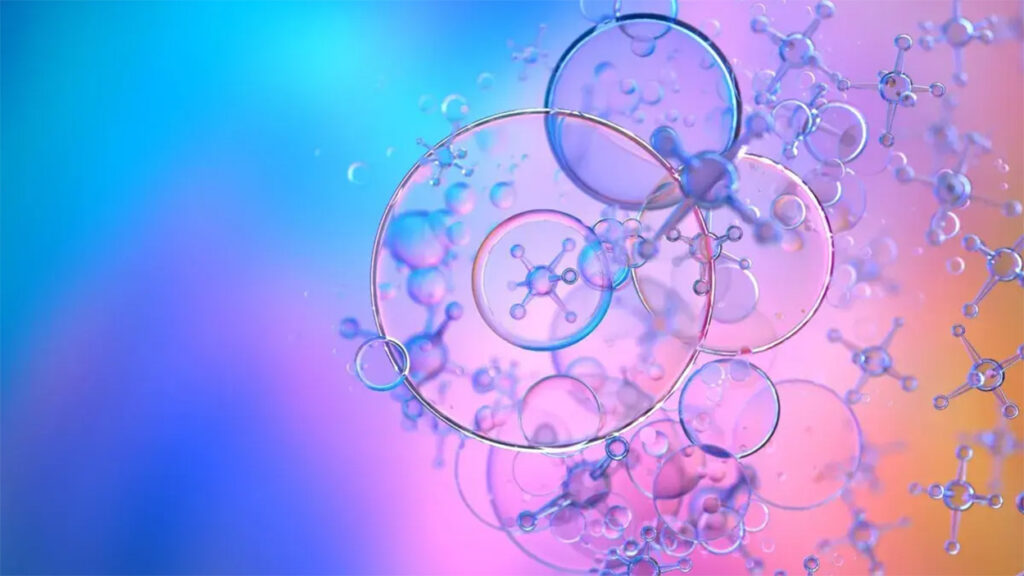
Training a machine to learn the language of molecules
The MIT team took a different approach to avoid the lengthy and costly period for drug discovery. Their machine-learning system automatically learns the language of molecules (molecular grammar) using a small dataset that is domain-specific. The system uses grammar to build viable molecules and simultaneously estimate their properties.
Molecular grammar is similar to language theory. A set of production rules tells the system how to generate the polymers or molecules by combining certain atoms and substructures, thereby creating many molecules. Since they train the machine to recognize the similarities, it becomes easier for the system to foretell the new molecules’ properties with a higher efficiency level. With a hierarchical approach, the learning process becomes faster.
Aside from making drug discovery faster, which will boost the medical community worldwide, the researchers want to extend their system to include 3D geometry of polymers and molecules to understand the interactions between polymer chains better.