When AI Eats Itself: The Perils of Synthetic Data Loops
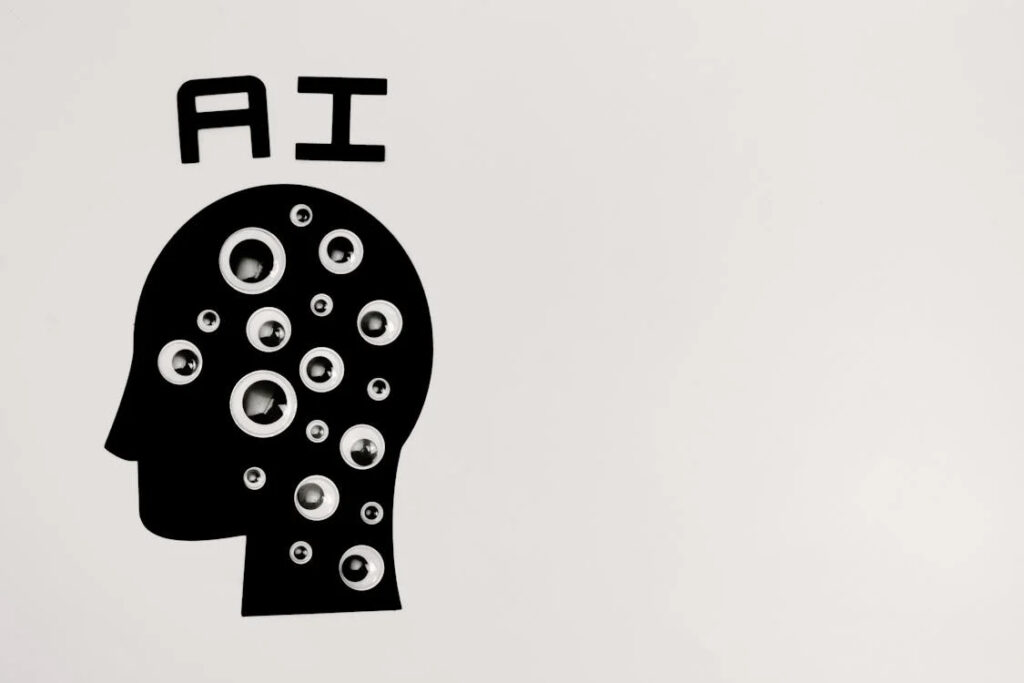
General artificial intelligence (AI) has transformed our production and interaction with digital material. From text to graphics, artificial intelligence models have apparently almost infinite creative possibilities. But as these algorithms get more sophisticated, the need for large-scale information to teach them is more pressing. Synthetic data is being used by developers in response to data shortage challenges. Although this method has great potential, it also brings major hazards that may compromise the basic basis of generative artificial intelligence.
The Synthetic Solution and Its Appeal
Synthetic data—created rather than acquired by direct measurement—offers a workable answer to the data scarcity issue in artificial intelligence training. Not only is this kind of data copious and reasonably priced, but it also provides a limitless pool from which artificial intelligence models may develop. Given the data does not match actual people, privacy issues—especially pertinent in sectors like healthcare—are lessened. Furthermore, synthetic data may be created to improve artificial intelligence performance by stressing particular traits or events that might not be well-represented in actual datasets.
The Downward Spiral of Data Degradation
Despite its initial benefits, the reliance on synthetic data can initiate a perilous feedback loop. When AI models are trained on outputs generated by previous iterations of themselves, the data can progressively lose touch with reality. This autophagous cycle, where models consume and regurgitate their own data, leads to a degradation of data quality over time. The phenomenon of ‘model collapse’ becomes a tangible risk, marked by a significant drop in the diversity and reliability of the AI-generated outputs. As models become increasingly self-referential, they may produce outputs that are not only less diverse but also bizarre and unusable in practical applications.
Amplified Risks in Critical Applications
Synthetic data loops provide actual hazards in important applications of artificial intelligence, not only odd or faulty digital artifacts. Where decision-making in fields like autonomous driving, medical diagnostics, or financial forecasting mostly depends on data integrity, the results may be disastrous. An artificial intelligence educated on low-quality synthetic data, for example, can misdiagnose a patient or misread financial patterns, resulting in real-world consequences that might weaken faith in AI technology.
The Need for Fresh Data Infusions
Maintaining a constant flow of fresh, real-world data into AI training cycles helps to reduce these hazards. This method guarantees that artificial intelligence models stay rooted in reality and that their outputs are still accurate and pertinent. Developers have to create procedures to strike a mix between synthetic and actual data, while observing how training tools affect model integrity and performance. Preserving the resilience of artificial intelligence systems depends critically on ensuring a variety of data sources and avoiding too strong dependence on synthetic inputs.
As AI continues to evolve, the strategies we employ to train these systems must also adapt. Unquestionably appealing and providing a shortcut to large, customized datasets, synthetic data is but the dangers of this method—especially the possibility of self-consuming data loops—demand a careful and measured approach. We can fully utilize artificial intelligence without falling victim to its own creating by including fresh, real-world data and building strict checks on data quality and variety. Solid data grounds should form the foundation of artificial intelligence as they combine integrity with innovation to really raise our technological capacity.