Reasoning Limits of AI: A Study on Cognitive Flaws in Large Language Models
The Inconsistency of AI Responses
Large Language Models (LLMs) like ChatGPT, which are at the heart of many generative AI platforms, have demonstrated significant advances in their ability to mimic human-like text, images, audio, and video outputs. However, a recent study from researchers at University College London (UCL) has highlighted a critical limitation in these models: their inconsistency in reasoning. The study employed standard cognitive psychology tests, such as the Wason task, the Linda problem, and the Monty Hall problem, to assess the reasoning capabilities of various LLMs. Despite their sophistication, these models often provided varying answers to the same questions and failed to show improvement even when additional context was provided. This inconsistency raises questions about the reliability of LLMs in roles that require stable and reliable decision-making.
Cognitive Challenges in Reasoning Tests
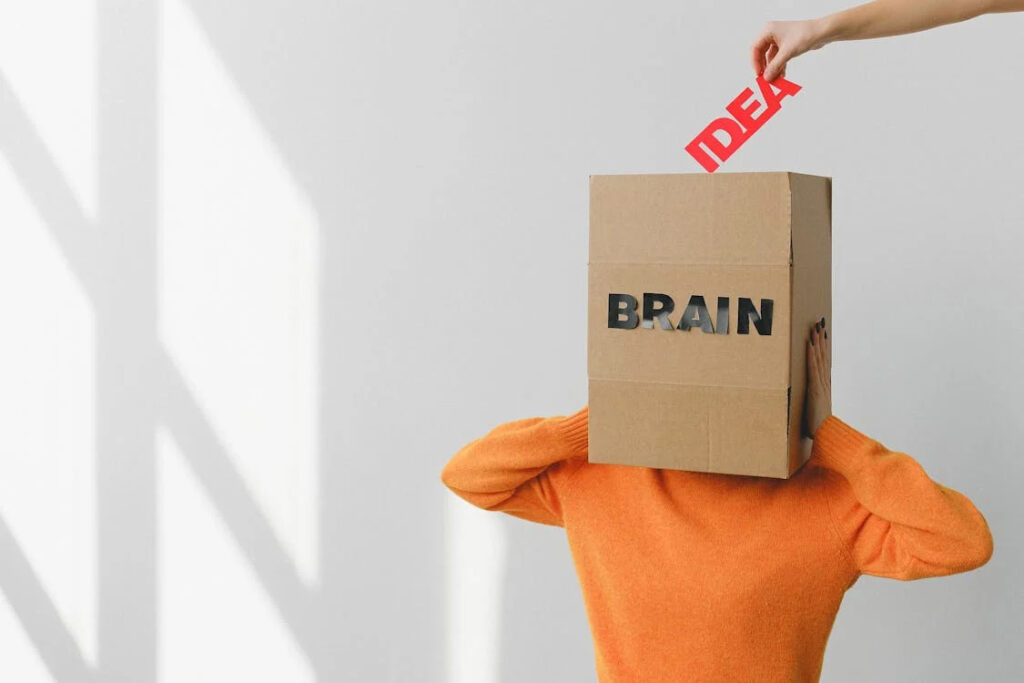
The UCL study revealed that LLMs struggle significantly with tasks that human participants typically find challenging. For instance, in cognitive tests like the Linda problem and the Wason task, only a small fraction of human subjects tends to provide the correct answers. Similarly, LLMs also performed poorly, but for reasons that differ from human challenges. For example, models like GPT-3.5 and Google Bard showed a wide range of errors, from basic arithmetic mistakes to misunderstanding letters as vowels—errors that are less likely to occur with human reasoning. These findings suggest that while LLMs can mimic the form of human intelligence, they lack a genuine understanding or the ability to apply logical and probabilistic rules consistently in their reasoning.
Data Volume and Model Performance
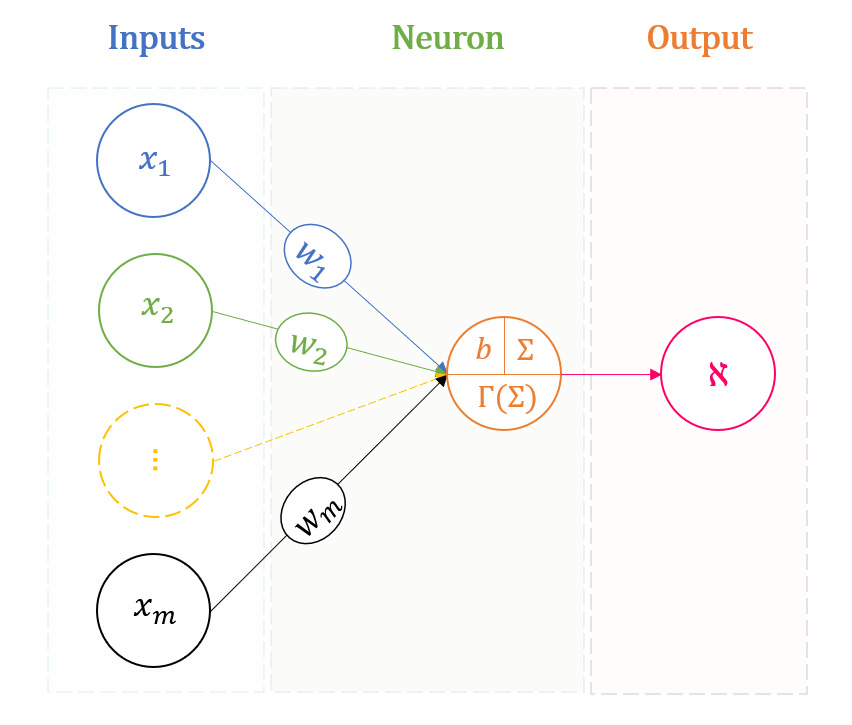
Among the models tested, GPT-4 stood out by showing better performance on several cognitive tests compared to its predecessors and other models like Google Bard and various versions of Llama. This improvement points to the influence of larger datasets and more advanced training techniques in enhancing model reasoning capabilities. However, the study’s lead author, Olivia Macmillan-Scott, pointed out that even with these advancements, it remains challenging to determine exactly how these models reason due to their ‘black box’ nature. This lack of transparency in the decision-making processes of LLMs is a significant hurdle in understanding and improving their reasoning capabilities.
Ethical Considerations and Future Directions
The UCL study also touched upon an interesting aspect of LLM behavior concerning ethical decision-making. Some models opted not to respond to certain tasks, citing ethical reasons, which could be a manifestation of the safeguarding parameters integrated into their programming. This behavior highlights the potential ethical dilemmas and biases that can be embedded within AI systems, consciously or unconsciously by their developers. Professor Mirco Musolesi, the senior author of the study, emphasized the need for a deeper exploration into the emergent behavior of these models. He suggested that understanding these aspects could lead to more refined methods of training LLMs that consider both the enhancement of their reasoning capabilities and the ethical implications of their use in real-world applications. This reflection on the limitations and potential biases of LLMs raises fundamental questions about the design of future AI systems—whether the goal should be to emulate human reasoning with all its imperfections or to strive for an idealized version of rationality.