Exploring the Limitations and Potential of AI in Wildlife Image Retrieval
In many different disciplines, artificial intelligence (AI) is becoming a useful tool; its application in biodiversity research is not an exception. Researchers are looking at how artificial intelligence systems—especially multimodal vision language models (VLMs)—may help to retrieve pertinent wildlife photos given the increasing volume of nature image databases. These models’ ability to retrieve photos from a large dataset was recently validated, so clarifying their strengths and constraints. The study provides understanding of how artificial intelligence might be used in environmental studies going forward. It also emphasizes the need of enhancing these systems to fulfill the particular requirements of biologists.
The Power of Multimodal Vision Language Models
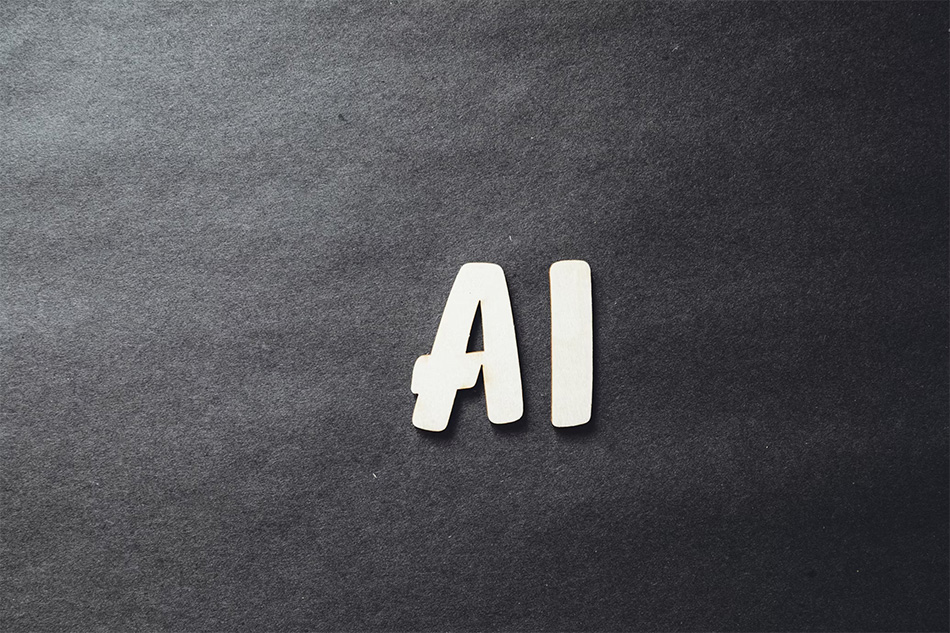
For nature researchers looking to examine large amounts of data, VLMs—trained on text and images—offer a potential fix. For ecological investigations, these models can help to simplify the search for particular images, including rare behaviors or unique biological conditions, which are absolutely essential. Though larger models struggled with more complicated questions requiring domain-specific knowledge, they did well on simple searches, suggesting the need of greater research. These models may greatly increase the efficiency of biodiversity study as they grow more sophisticated. Their fast sifting of massive data could hasten the finding of important new ideas about animals.
Challenges in Processing Complex Scientific Queries
The research underlined a major difficulty: When assigned more complex searches, such spotting particular biological conditions or unique behaviors, VLMs typically stumbled. For instance, the models battled more technical questions like seeing axanthism in frogs while they could recognize jellyfish on a seashore. This implies that to completely understand and handle difficult scientific language and ideas, VLMs need more specific training data. The challenge of converting intricate biological words into a format AI can efficiently understand reflects their present discrepancy in capability. Making VLMs more valuable in advanced ecological research depends on addressing these difficulties.
The Role of the INQUIRE Dataset
Using the INQUIRE dataset—five million animal photos and 250 search questions from ecologists and other biodiversity experts—the researchers assessed the performance of the VLMs. This dataset was created to mirror the kinds of particular, rare events that pique the curiosity of academics, such a hermit crab with plastic trash as its shell. Notwithstanding its volume and importance, the dataset exposed flaws in present artificial intelligence models, especially in terms of interpreting sophisticated scientific language and challenging questions.
Future Prospects for AI in Biodiversity Research
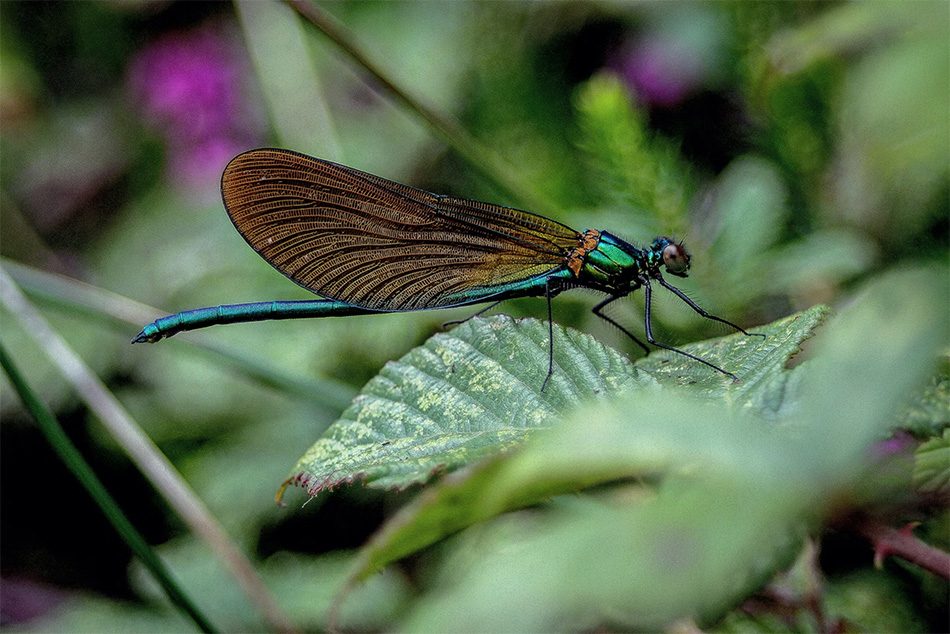
Although present VLMs are not yet completely capable of managing challenging searches, research indicates a bright future. These models might be quite helpful research partners for ecologists, biologists, and other environmental scientists given more domain-specific data and improved algorithms. Eventually, the continuous evolution of systems such as the one shown by the researchers could enable scientists more effectively extract the precise photos they require, hence improving biodiversity monitoring and conservation initiatives.
Although artificial intelligence is still in its early years and has great difficulties to be overcome in relation to biodiversity study. Current VLMs struggle with more complicated scientific searches even if they can help with simpler inquiries. Still, their potential to transform the area of wildlife picture retrieval is great as the models develop and acquire access to more specialized training data. With the possibility to significantly improve the accuracy and efficiency of biodiversity monitoring, artificial intelligence in ecology seems to have bright future. As long as we keep developing, artificial intelligence may be indispensable in preserving the diversity of the earth. Its ability to examine enormous volumes of data may bring vital new perspectives on the state of ecosystems all around.