Enhancing Equity in AI-Driven Mental Health Support
Artificial intelligence (AI) has great promise to solve mental health issues but also complexity as it gets more and more included into healthcare. AI-powered chatbots—such as those built on big language models (LLMs) like GPT-4—are under investigation as means to increase mental health support availability. Recent studies, however, draw attention to important problems, especially with relation to equity and bias in these systems, which calls into doubt their dependability in different clinical settings. This emphasizes the need of a better knowledge of the operation of these instruments. AI systems must be maximized for inclusivity, hence stakeholders have to guarantee that. Estaining confidence in AI-driven healthcare solutions depends on addressing these obstacles.
The Growing Role of AI in Mental Health
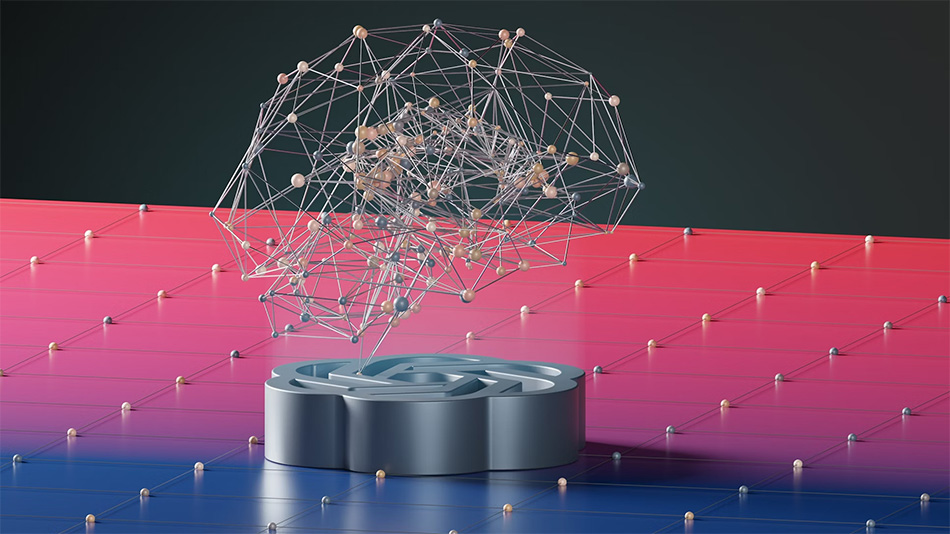
Given millions of people lack access to conventional therapy, artificial intelligence chatbots are considered as a remedy for the dearth of mental health specialists. These systems seek to fill care gaps by means of user inputs and sympathetic reactions. Studies reveal that replies produced by artificial intelligence sometimes show more empathy and are more adept than human ones at motivating favorable action. These developments however highlight the need of careful application given the possibility of negative consequences such improper advice or strengthened vulnerabilities. Minimizing risks depends on strong safety procedures being developed. More effective tools result from the cooperation between mental health professionals and artificial intelligence engineers. Their dependability will be even improved by constant monitoring and feedback loops.
Evaluating Empathy and Bias
Studies have compared the empathy levels of artificial intelligence and human reactions to mental health searches using datasets from sites like Reddit. Although GPT-4 generally showed more broad empathy than human responses, its performance differed depending on demographic groupings. For posts from Black and Asian people, for instance, reactions were routinely less sympathetic than those for White people or those with unidentified demographics. This difference emphasizes how both explicit and implicit demographic factors influence artificial intelligence behavior. Dealing with these differences calls for algorithmic tweaks and focused interventions. In model building, researchers have to give justice and equity first priority. Greater responsibility will result from more transparent evaluation systems.
Addressing Bias in AI Systems
Directing LLMs explicitly to take demographic factors into account has demonstrated to lower their responses’ bias. Researchers have seen increases in fair empathy levels across several groups by customizing prompts and offering context-specific instructions. This method underlines the need of deliberate design and continuous assessment in order to reduce the biases in artificial intelligence systems. Frequent evaluations of artificial intelligence systems help to find and fix possible prejudices. Including several user groups in testing stages improves diversity. Setting ethical guidelines for artificial intelligence uses should also be a responsibility of policy makers.
Implications for Clinical Applications
Using artificial intelligence in clinical environments calls for thorough testing to guarantee fair support for every user. Emphasizing their effectiveness across several demographic categories, researchers support thorough systems to assess artificial intelligence tools. Such initiatives are crucial to avoid unanticipated effects and to foster confidence in mental health treatments powered by artificial intelligence. Maintaining user privacy and data security comes equally first. Integration might be improved by teaching doctors on artificial intelligence techniques. Public awareness initiatives can assist to demystify the function of artificial intelligence in mental health treatment.
By improving access and raising support, artificial intelligence could transform mental health treatment. But reaching fair and consistent results requires overcoming prejudices and improving these systems by careful design and review. The objective of study is still to make sure that, independent of background, AI tools offer compassionate and objective treatment for every person. Improving multidisciplinary cooperation will help this field to advance faster. Clearly defined rules for ethical AI application are absolutely necessary. The effectiveness of artificial intelligence in mental health ultimately depends on its capacity to efficiently service many groups.