Human Workers Can Become Lazier When Working with Robots and AI
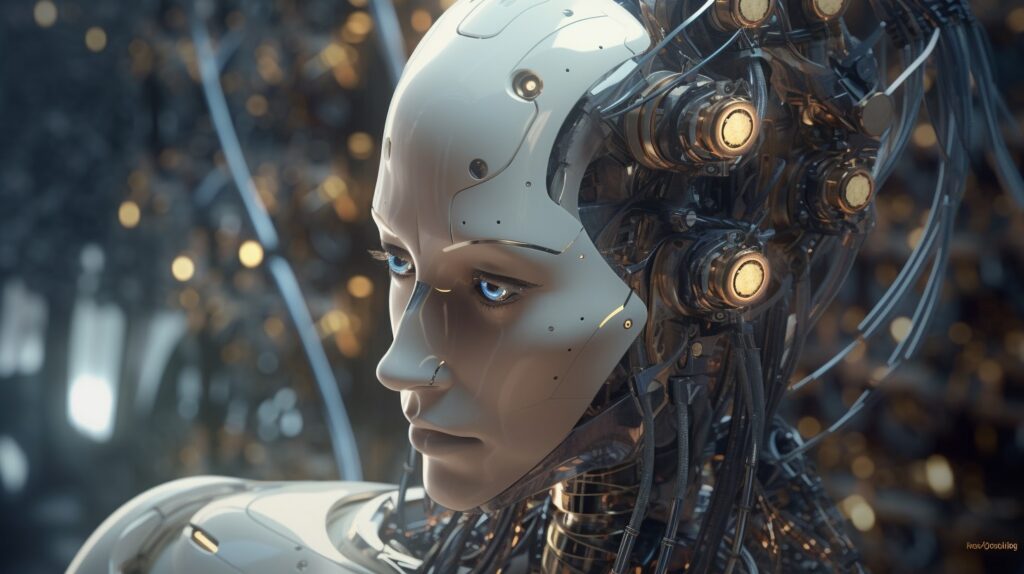
With technological breakthroughs enabling robots to work alongside humans, there is evidence that individuals are starting to see these robots as teammates. Teamwork can have both positive and negative consequences on a person’s performance. People could become complacent and let their human or robotic coworkers take on most tasks.
People frequently engage in “social loafing” when they know that their contributions will go unappreciated or when they have grown accustomed to another team member’s high level of performance. Researchers at the Technical University of Berlin looked into the possibility of social loafing by humans using robots.
Dietlind Helene Cymek, the study’s first author, who recently had her findings published in the journal Frontiers in Robotics and AI, remarked that teamwork is a mixed blessing. Working together can inspire people to deliver their best work but also cause them to lose enthusiasm because each person’s contribution is less obvious. Her team was curious if they could detect similar motivational effects when the teammate is a robot.
Getting help
The researchers tested their theory by doing a mock industrial defect inspection work, which involved checking circuit boards for flaws. Forty-two participants received pictures of circuit boards from the researchers. Circuit boards were hazy, and the sharpened images were only visible by hovering a mouse tool over them. The scientists could then monitor how closely each person examined the board.
Half of the participants were informed that a Panda robot had just inspected the circuit boards they were working on. Although these individuals weren’t directly involved with Panda, they could hear and see it while working. All participants were asked to rate their effort, how accountable they felt for the assignment, and how they did after checking the boards for faults and marking them.
The time spent reviewing the circuit boards and the area searched did not appear to differ statistically significantly across the groups, giving the impression that Panda’s presence had made no difference. Participants in both groups gave identical ratings of their sense of accountability for the work, their level of effort, and their performance.
Looking versus seeing
The participants using Panda were catching fewer errors later in the exercise when they had already seen that Panda had successfully detected many errors. The researchers discovered this after paying closer attention to participant error rates. This might demonstrate the “looking but not seeing” effect, in which people become accustomed to depending on something and give it less of their mental attention. Although they said they were paying equal attention, the participants unconsciously believed that Panda hadn’t missed any errors.
According to Dr. Linda Onnasch, the study’s principal author, it was easy to track where a person was looking. However, it is more difficult to tell whether the visual information is adequately processed mentally.
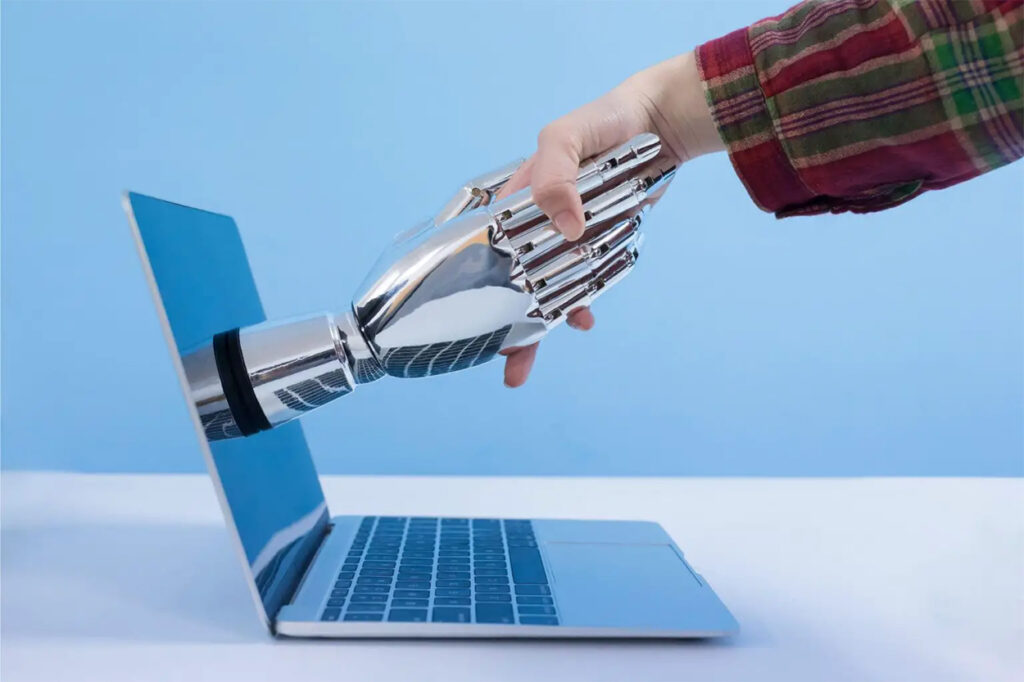
Safety risk
The authors expressed concern that this might have safety repercussions. Dr. Onnasch said that the subjects worked on the task for about 90 minutes in their experiment. They found fewer quality errors were detected when working in a team. The loss of motivation tends to be considerably larger during longer shifts. The duties are more routine during this time, and the work environment provides little performance monitoring and feedback. Thus, this can have a negative effect on job outputs in manufacturing generally. Moreover, this can significantly affect safety-related areas where double-checking is widespread.
The researchers emphasized the limitations of their test. The participants did not work directly with Panda despite being told they were part of a team with the robot and seeing some of its work. Additionally, because participants are aware of being observed, social loafing is challenging to replicate in a lab setting.
The laboratory environment, according to Cymek, is the primary constraint. They want to go to the field and test their hypotheses with skilled workers who regularly work in teams with robots. They want to discover the extent of the problem and loss of motivation in human-robot interaction settings.